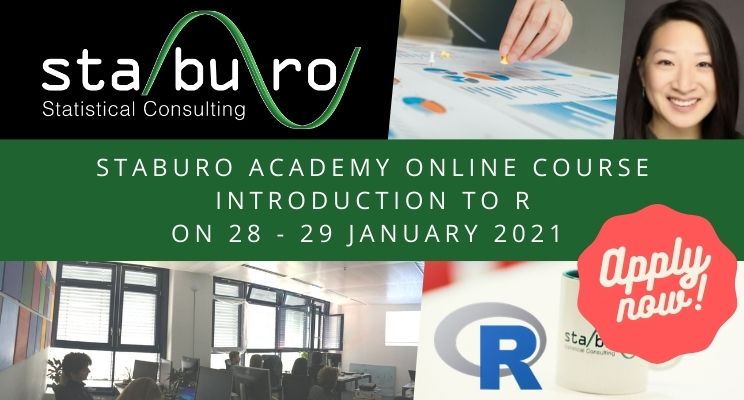
Staburo Academy online course Introduction to R
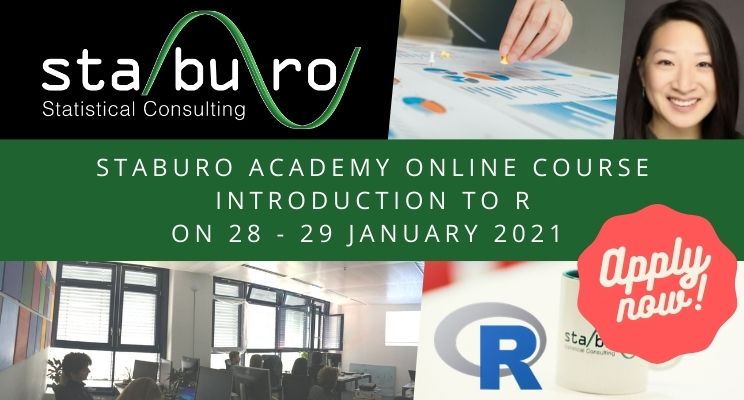
Introduction to R online course
Our course “Introduction to R course” will be a 2-day course from 28 to 29 January 2021!
The course, which takes place online, is about providing a solid foundation in R, the popular open source programming language in statistics and data sciences. As a preferred tool for complex data analyses it is applied in every industry. This R programming course will give an introduction to the basics of R. In the two-day course you will learn how to program in R, how to perform data analysis in R and how to visualize your results graphically.
The course is mainly addressed (but not restricted) to professionals who are interested in data science (e.g. Statisticians, SAS Programmers, Data Managers) and who want to learn the very basics of R. Further, this introduction is helpful for participants who wish to brush up on their knowledge in R.
The course will be held in English and the detailed agenda will be sent out a few days before the start of the course. You find more details here!
Recent Comments