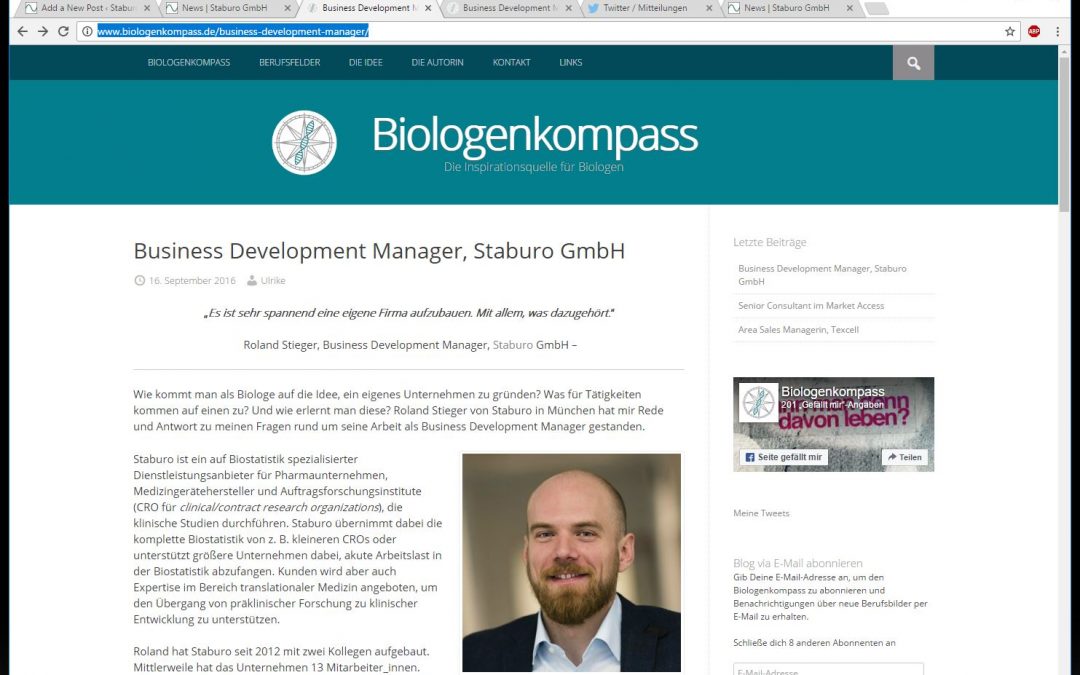
Story about our Business Development Manager Roland Stieger on biologenkompass.de
The website biologenkompass.de published a story about our Business Development Manager Roland Stieger (in German).
The interview was done by Ulrike Herzog, who is one of the founders of biologenkompass.de. The goal of her team is to inform biologists with potential job opportunities after their Master’s degree or PhD thesis.
We are very happy to support them in their project and thank biologenkompass.de for the interview!
Recent Comments